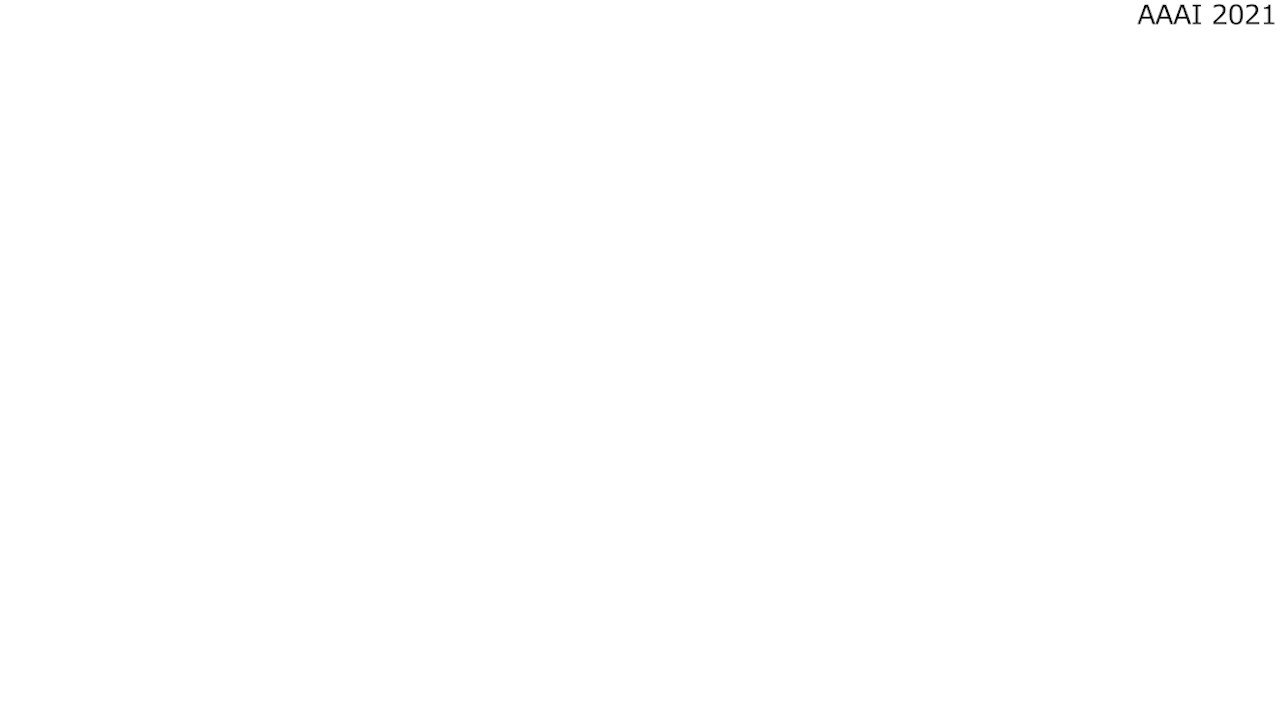
@inproceedings{Kim2021FixMyPosePC,
title={FixMyPose: Pose Correctional Captioning and Retrieval},
author={Hyounghun Kim and Abhaysinh Zala and Graham Burri and M. Bansal},
booktitle={AAAI},
year={2021}
}
Rank | Model | Code | Language | Automated Metrics | Task-Specific Metrics | ||||||
---|---|---|---|---|---|---|---|---|---|---|---|
B4 | C | M | R | OM | BM | DM | |||||
1
September 9th, 2020 |
Baseline Model
UNC Chapel Hill (Kim, Zala, et al. 2020) |
Github | English | 17.26 | 6.40 | 21.30 | 34.82 | 0.04 | 1.42 | 0.17 | |
Hindi | 18.98 | 6.69 | 28.47 | 34.53 | 0.03 | 1.52 | 0.11 |
Rank | Model | Code | Accuracy (%) | |
---|---|---|---|---|
English | Hindi | |||
1
September 9th, 2020 |
Baseline Model
UNC Chapel Hill (Kim, Zala, et al. 2020) |
Github | 38.49 | 37.84 |